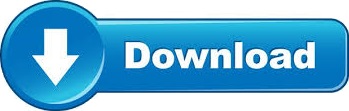

Keep in mind that if you use specialized statistics software (e.g., R or Python libraries), you won’t have to create the dummy variables, as these frameworks recognize the quarter as a categorical variable. The period (t) variable will be the fourth variable in the regression model and will represent our time series. We will have three dummy variables (n-1) for Q1, Q2, and Q3, while Q4 will remain our baseline. We are going to treat every quarter as a separate dummy variable. To capture both the seasonality and potential underlying trend in the data, we will rely on the regression analysis functionality that is part of Excel. The last quarter of the year is where the company performs best in terms of generating sales revenue. We can calculate these averages with the AVERAGEIFS function in Excel: Some years show a more prominent jump in sales revenue than others, but we can safely conclude there’s some seasonality in the data series.Īnother way to test for seasonality is to calculate the average sales revenue for each quarter in the different years. As soon as we look at the line chart above, we notice some spikes in Q4. One way to detect seasonality is by visually examining the data.
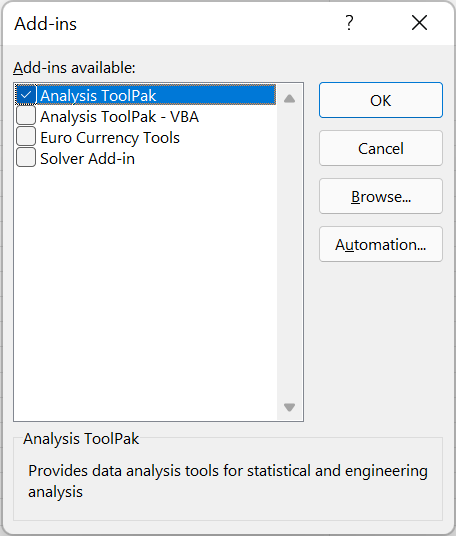
If we add the quarters to the data and plot it on a simple line chart we get: The aim is to create a model that can help us forecast the revenue of GoPro for the next financial year, 2020. We have the data for the period 2013 to 2019. Let’s look at the quarterly sales revenue of the electronic cameras manufacturer GoPro (source: ). Today we will use regression analysis in Excel to forecast a data set with both seasonality and trend. We went over an example Excel model of calculating a forecast with seasonality indexes. In our last article, we discussed Seasonality in Financial Modeling and Analysis.
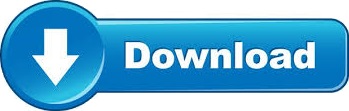